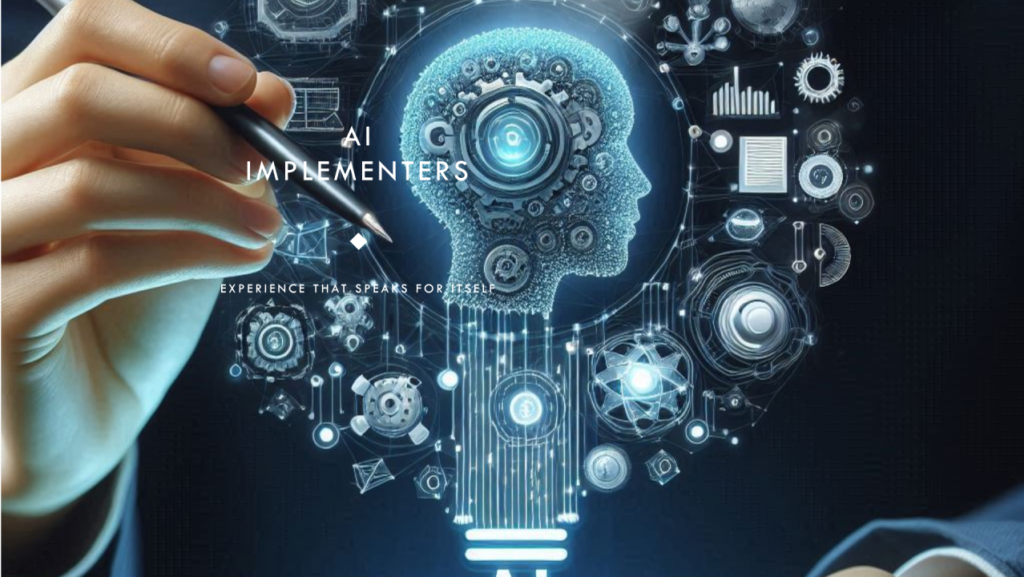
This article was initially published by the Institute for Artificial Intelligence and Big Data (AIBIG) in AI Spectrum Edition 2.
Artificial Intelligence (AI) stands at the forefront of modern technological advancements, promising to revolutionize industries and societies. However, the journey from theory to practical application often reveals a significant gap – one that challenges academic researchers, developers, industries and policymakers.
In theory, AI involves various disciplines such as computer science, mathematics, biology, and more. The primary objective in the fundamentals and theory of AI is its capability to learn from data, make decisions, and autonomously solve complex problems. All the AI technologies we see today derive from scientific breakthroughs made by academics and researchers in higher education. Indeed, it is not an overnight process; it took decades for scientists to achieve new findings that we now embrace today.
In the past, most major AI research was primarily conducted in universities, such as the development of machine learning models. This was because the algorithms and methods developed in academia needed extensive experiments. However, since 2014, the industry has taken over. Building machine learning models quickly requires large amounts of data, computing power, and funding—resources that are more readily available in industry compared to academia.
However, the application of AI in practice reveals a complex landscape. While progress in machine learning algorithms, neural networks, and deep learning has boosted AI’s capabilities, deploying these technologies effectively requires addressing challenges such as data privacy, ethical considerations, and societal impacts. The gap between AI theory and practice highlights the importance of interdisciplinary collaboration and strong ethical guidelines. Building AI applications requires diverse expertise. For example, if we want to develop AI applications in healthcare, we need medical experts who can validate genetic biomarkers predicted by deep learning algorithms.
In practice, AI appears in various forms—from chatbots automating customer service to sophisticated AI systems aiding in medical diagnostics. Each application brings its own set of challenges and opportunities, demanding ongoing improvement and adaptation. The evolution of AI applications depends on addressing real world complexities while harnessing the potential for transformative innovation responsibly.
As we navigate this dynamic landscape, bridging the theory application gap requires ongoing dialogue, research, and a commitment to ethical AI development. By aligning theoretical insights with practical realities, we can unlock AI’s full potential to benefit humanity while addressing its challenges head on. However, the question now arises: What are our capabilities, and how ready are we to develop the talents, solutions, and scientific breakthroughs in AI that have largely been neglected by our academic researchers? Commitment, continuous exploration, and innovation must be our focus to shape our technological future.
“The gap between AI theory and practice highlights the importance of interdisciplinary collaboration and strong ethical guidelines.”